Education |
FRM
The Role of Artificial Intelligence (AI) in Financial Risk Management
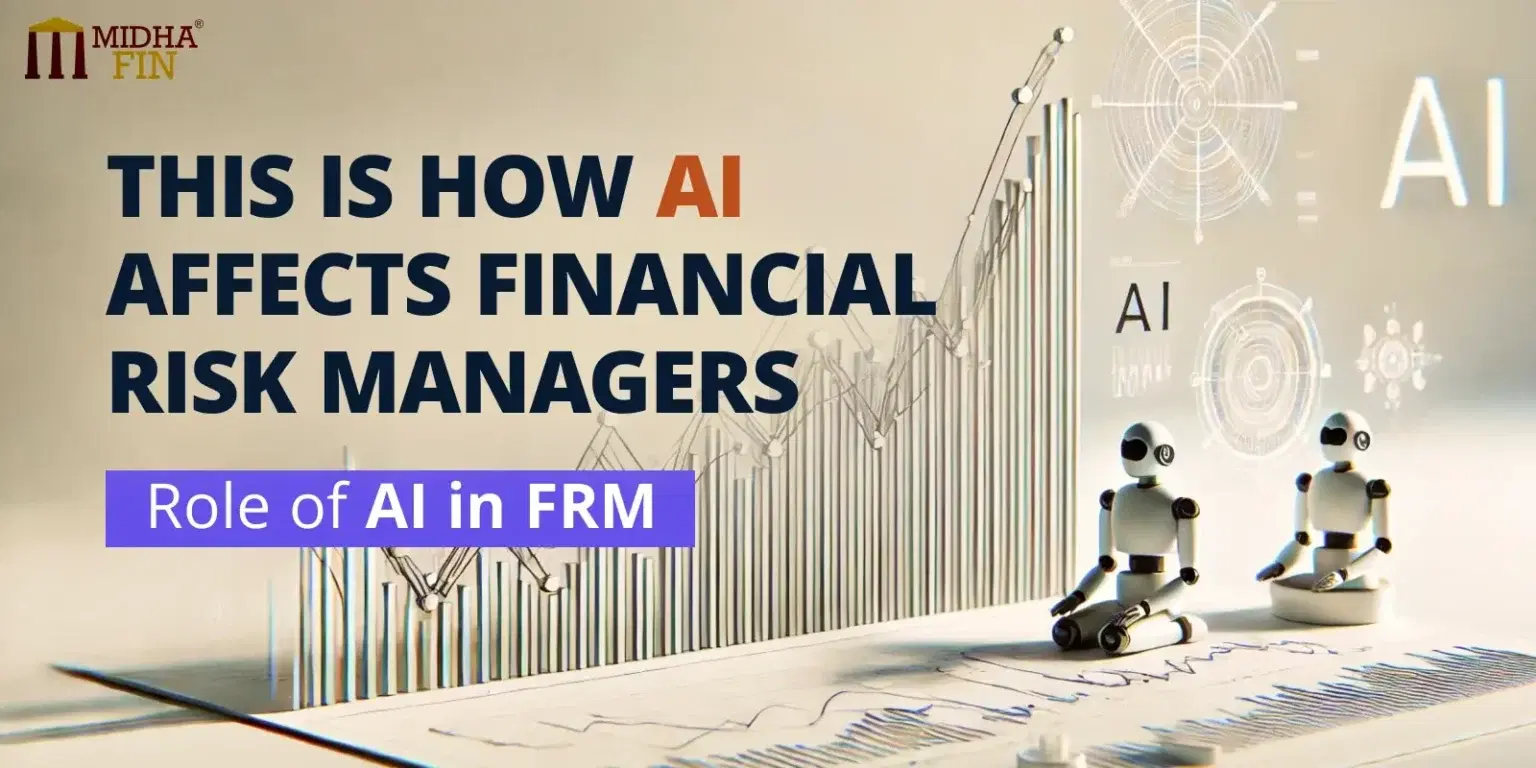
In the modern dynamic financial markets, traditional approaches to risk management turn out to be hardly applicable. Mostly expensive and time-consuming, conventional methods do not correspond to the demanding time frames, a common feature of contemporary finance. That is precisely where artificial intelligence comes into play, offering new ways and opportunities for enhancing financial risk management processes. AI in the financial sector is not just another technology; it is changing the entire scenario regarding risk management. The successful adoption of AI into operational activities by financial institutions has further made better decisions in conducting business with greater precision in predicting and mitigating risks.
AI in Credit Risk Management
Credit risk management, which analyzes the likelihood of a counterparty defaulting on its commitments, is pivotal to financial stability. Traditionally, these models have been statistical methods, but their effectiveness is now being diminished because of lightning changes in fintech. AI, particularly machine learning (ML), offers a more advanced approach to credit risk modeling and assessment. Techniques of machine learning include support vector machines and decision trees and greatly improve models of credit risk by revealing patterns and anomalies in large datasets. This ability helps lending institutions refine their credit-scoring systems and make better judgment calls. For example, deep reinforcement learning can further sharpen the feature selection related to, but not limited to, credit risk management. In addition, using AI builds accurate credit-rating profiles and, therefore, improves access to credit among startups and small businesses. There is also the use of AI in credit risk management to reduce default rates and improve overall financial stability.
AI in Market Risk Management
Market risk stands for the risk a financial entity faces concerning possible loss arising out of market price fluctuations, such as commodities, interest rates, and foreign exchange rate variations. Modern advanced tools are available that can predict and mitigate market volatility. ML, more precisely, ML techniques in market risk management, helps in data preparation, model validation, stress testing, and risk modeling. This is because machine learning algorithms can handle raw data volumes of the markets and hence provide more accurate risk assessment. For instance, AI can allow stress testing on market models to find non-intended risks and strategic feedback. Financial institutions use AI to fine-tune trading strategies, evaluate Value-at-Risk, and forecast possible shortfalls. Using AI in market risk management, institutions are assured of accuracy and savings on operational costs, which means the institution maintains competitiveness and is resilient.
AI in Operational Risk Management
This is the kind of risk in which losses can result from failures in the internal processes or systems or due to external events. This includes risks from fraud, human errors, and technological breakdowns. AI greatly enhances ORM by automating repetitive tasks, improving data quality, and detecting fraudulent activities. It can explore large amounts of data for the identification of operational risks and make a suggestion for mitigation strategies by machine-learning algorithms. For instance, AI can facilitate the process of detecting any kind of abnormalities within the financial transaction being made, thereby reducing fraud risks. This will also help support the decision-making process by providing complete and accurate information to the risk manager. Investing in AI for ORM makes an organization competitive and efficient in dealing with operational challenges. This frees businesses from labor-intensive procedures and allows them to concentrate on the strategic management of risks and future growth.
Challenges in Use and Future Directions for Research
Despite these many advantages in FRM, there are a number of challenges to the adoption of AI technology. This includes the need for high-quality data, the complexity of implementing an AI system, and regulatory concerns. Future research and development should overcome these challenges. This is by enhancing data management practices, transparent models of AI, and keeping regulatory standards. Adopting AI in FRM is critical as the financial market transforms continuously. AI can be used to transform financial risk management by providing more reliable predictions at low cost with increased stability.
Conclusion
AI’s impact on financial risk management is significant. Artificial intelligence techniques will help financial institutions advance their risk management strategies, take better decisions, and continue to compete in the changing market. The future of FRM relies on amalgamating the traditional methods with more advanced AI technologies that shall pave the way for a stronger and efficient financial system.