Risk Management
Testing VaR Models
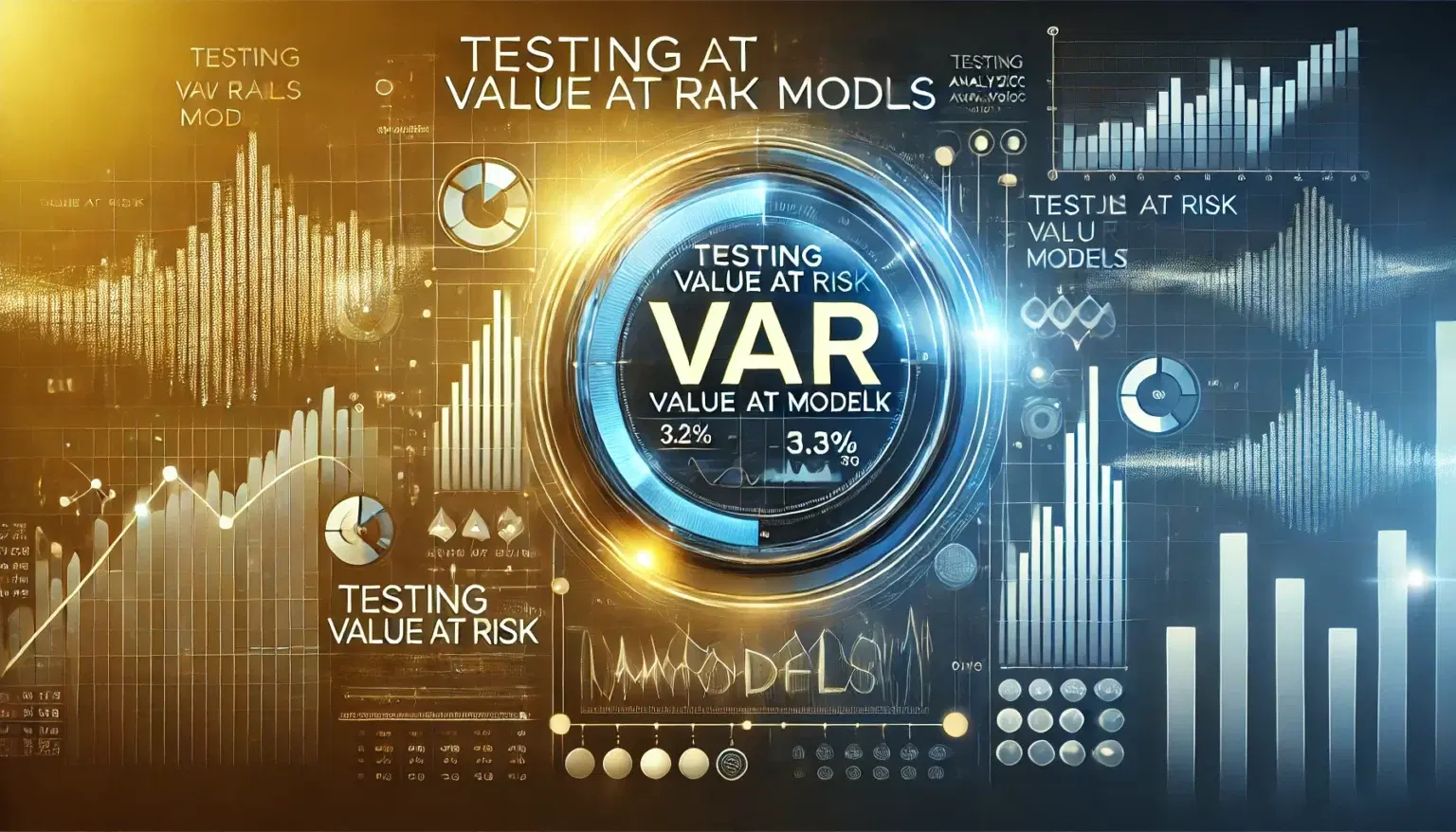
How Banks Test Their Value at Risk (VaR) Models
Value at Risk (VaR) is a cornerstone of modern financial risk management. This statistical technique estimates the potential loss in value of a portfolio over a specified time frame and confidence interval. While widely used, ensuring the accuracy and reliability of a VaR model is paramount for banks to effectively manage risk and comply with regulatory requirements. To achieve this, banks employ rigorous testing methodologies to validate and improve their VaR models. Here is an overview of the most common approaches used to test VaR models.
1. Backtesting
Backtesting is a fundamental technique used to assess the accuracy of VaR models. The process involves comparing the model’s predictions against actual portfolio performance. Using historical data, banks determine how often actual losses exceed the predicted VaR (referred to as exceptions).
To guide this evaluation, regulatory frameworks such as Basel II/III employ a “Traffic Light Approach”:
- Green Zone: Few exceptions, indicating the model is reliable.
- Yellow Zone: Moderate exceptions, requiring closer monitoring.
- Red Zone: Excessive exceptions, signaling potential underestimation of risk.
Backtesting provides direct feedback on the model’s ability to predict potential losses, offering a critical measure of its reliability.
2. Stress Testing
Stress testing complements VaR by evaluating the model’s performance under extreme market conditions. This technique involves simulating portfolio losses during hypothetical or historical crisis scenarios, such as financial crashes or sharp interest rate changes.
Key objectives of stress testing include:
- Identifying vulnerabilities in the portfolio.
- Ensuring the model can account for risks outside normal market conditions.
By testing extreme but plausible scenarios, banks gain confidence in the model’s ability to manage tail risks.
3. Sensitivity Analysis
Sensitivity analysis assesses how the VaR model responds to changes in its assumptions and inputs. By adjusting variables such as volatility, correlations, or holding periods, banks can identify how sensitive the model is to these parameters.
This process helps highlight any over-reliance on specific assumptions and ensures that small changes in inputs do not lead to disproportionately large deviations in risk estimates.
4. Monte Carlo Simulations
Monte Carlo simulations are a robust technique for testing VaR models under a wide range of scenarios. This method involves generating thousands (or millions) of random price paths for portfolio assets based on probabilistic distributions.
By calculating potential losses across these scenarios, banks can:
- Validate the accuracy of their VaR estimates.
- Evaluate tail risks and loss distributions comprehensively.
Monte Carlo simulations are particularly effective in capturing complex portfolio dynamics and non-linear risks.
5. Benchmark Comparisons
Benchmark comparisons involve testing the VaR model against alternative risk measures or external models. For instance, comparing VaR estimates to those from:
- Expected Shortfall (ES): A metric that considers average losses beyond the VaR threshold.
- GARCH Models: Used to estimate time-varying volatility.
This approach identifies inconsistencies and validates whether the VaR model aligns with other risk assessment tools.
6. Hypothesis Testing
Statistical hypothesis tests are employed to rigorously evaluate the model’s predictive accuracy. Commonly used tests include:
- Kupiec Proportion of Failures Test: Assesses whether the observed exceptions match the expected frequency for the chosen confidence level.
- Christoffersen Test: Evaluates whether exceptions are independent and randomly distributed over time.
These tests help determine if the model’s predictions are statistically valid.
7. Regulatory Stress Tests
Banks must also conduct regulatory stress tests to comply with frameworks such as Basel III and the Dodd-Frank Act. These tests often involve:
- Applying scenarios provided by regulatory authorities.
- Demonstrating the adequacy of the VaR model in managing risks under adverse conditions.
Regulatory stress tests ensure that the model meets industry standards and provides an added layer of assurance to stakeholders.
8. Validation by Independent Units
To eliminate bias, independent validation teams or external auditors are tasked with assessing the VaR model. This validation process includes:
- Reviewing model assumptions, methodologies, and data inputs.
- Conducting additional tests to corroborate findings.
Independent validation ensures an objective evaluation of the model’s reliability.
9. Periodic Model Updates
Market conditions and portfolio compositions evolve over time, necessitating regular updates to the VaR model. Periodic recalibration involves:
- Incorporating updated market data.
- Revising assumptions to reflect current market realities.
Ongoing updates ensure that the model remains relevant and effective in capturing changing risk dynamics.
Challenges in Testing VaR Models
While these testing methodologies enhance the reliability of VaR models, challenges remain:
- Fat Tail Risks: VaR often struggles to capture extreme losses in the tail of the distribution.
- Non-Stationary Markets: Changing market dynamics can erode model accuracy.
- Correlations: Simplified assumptions about asset correlations may underestimate interconnected risks.
By addressing these limitations through robust testing, banks can improve their risk management frameworks and safeguard their portfolios.
Conclusion
Testing Value at Risk models is a critical aspect of risk management in the banking sector. Through a combination of backtesting, stress testing, sensitivity analysis, and other advanced techniques, banks ensure their models provide accurate and reliable risk assessments. These efforts not only help mitigate financial risks but also build trust among regulators and stakeholders, fortifying the resilience of financial institutions in an ever-changing market environment.